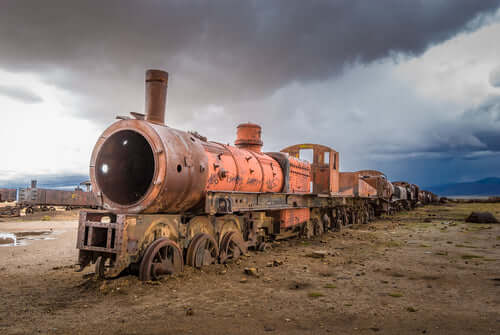
LegalTech Trainwrecks #7 (Tracking Knowledge Resources)
Over the past three years, I’ve prototyped and discarded about a dozen potential legaltech products. This is part 7 in a series of post-mortems on these failed projects. Follow the link to find the previous instalments of legal tech trainwrecks.
The problem
Fresh from the trainwreck that was my automated knowledge delivery system, I decided to tackle the problem of improving awareness and utilisation of law firm knowledge resources from a different angle.
Rather than trying to help lawyers directly by automatically serving them knowledge resources as they worked (it turned out this would mostly just generate a whole lot of unwanted noise), could I instead focus on helping the knowledge managers whose job it is to disseminate those resources?
Through my earlier interviews with knowledge managers, I learned that one of their biggest challenges was the battle for attention. One knowledge manager told me how they just had to ‘keep slogging it’ with communications. While I could sympathise with this, I did wonder whether the best way of getting the attention of busy lawyers, whose inboxes are already filled to the brim, was to send them more emails.
Taking a leaf from marketing practices, could I help knowledge managers devise a more nuanced approach if they had better data about what emails were being read, what training sessions were being attended, and what resources were being viewed?
Knowledge managers told me they didn’t currently do much of this. I asked why.
There was a little bit of, “It’s not within my job description”, and a little bit of, “I don’t think lawyers would want to be monitored like that”. But it was mostly, “the data is too hard to get”.
One knowledge manager described how fiddly it was to set up pageview metrics on a SharePoint page that housed knowledge material. Another told me they could track who had opened a particular knowledge resource stored on their DMS (document management system), but the process was very clunky. This was only the tip of the iceberg because other important knowledge distribution channels went completely untracked, such as email opens, attachment opens, or where lawyers had downloaded knowledge materials and were viewing them locally.
I asked whether getting their hands on this data would be useful. The response was, “Yes, definitely”.
It was time to test a solution.
The prototype
I hacked together a prototype and installed it on my own device. It could send usage data relating to specified knowledge resources back to a central dashboard. For example, if a knowledge team sent an email update regarding a law change, the prototype could track whether the lawyer opened that email and for how long, whether the lawyer opened any attached document and for how long, and even where the lawyer opened that document directly from SharePoint, the DMS, or their local drive.
So what did people think?
Well, having been presented with the prototype’s data, it began to dawn on knowledge managers that the data was perhaps not as useful as they had initially envisaged.
For instance, my prototype’s dashboard indicated a lawyer had heavily used a particular resource. But why was this the case? Was it because the lawyer had to read it over and over again because it was so badly written, was it because the lawyer had left it open on their screen while they went to the staff kitchen, or was it because the lawyer genuinely found it useful?
Similarly, where the dashboard indicated a lawyer had not used a resource very much, was it because the lawyer didn’t know about the resource, was it because the lawyer knew about the resource but felt they were already on top of that topic, so they didn’t need to use it, or was it because the resource was about something that didn’t come up very much in practice?
The list goes on. Unfortunately, the only way to get the answers to these important questions is to talk to the lawyers themselves, which knowledge managers already did or at least knew they should do. My prototype demonstrated that quantitative data could be obtained, but in the end, that exercise wouldn’t actually solve the underlying problem.
Déjà vu
The whole experience reminded me of a similar experiment I had conducted many years before while practising as a finance lawyer.
I had identified a problem around not having structured data on the provisions in contracts we had previously negotiated for our clients. The lack of structured data meant it was a manual task each time to find and read those previous documents to identify data points relevant to the current negotiations. For example, if I was working on a facility (credit) agreement, I might want to check the representations and warranties (each of those being a data point) in that agreement against the equivalent ones in previous facility agreements I had worked on.
To test a solution, I manually populated an Excel spreadsheet containing data points from 5 or 6 facility agreements, together with matter details such as deal size, industry, and parties. Given that it was a prototype, it was a small dataset, but it could still produce impressive-looking pie charts and bar graphs, tell me what the ‘market’ was, and show how different parties had approached different issues.
Yet despite putting all that effort into creating this myself, I distinctly remember using it precisely zero times.
Yes, it had a lot of data. No, that data was not actually useful.
I discovered that when comparing a current document against past documents, it was important for me to look at the exact wording from those past documents, as opposed to a summary represented by data points in a spreadsheet. Even though I had prepared the spreadsheet myself, I didn’t feel I could rely on it without checking there wasn’t some nuance in the original wording or something particular to the previous matter that perhaps didn’t seem relevant when creating the spreadsheet but would be important for the matter I was working on. Nor was it hard for me to access those past documents and find the relevant clauses. In most cases, those documents were already saved to my desktop, and by this stage, I knew my way around a facility agreement pretty well.
The idea of having lots of data and being able to extract insights from that data is seductive. It’s only when you try to implement it that you may realise the benefits are not as great as you hoped and the time and expense are more than you anticipated. Of course, sometimes the data will genuinely be useful and worth the effort of collecting, curating and analysing. But it’s not always the case. Sometimes having that data just doesn’t make that much difference.
Daniel Yim writes and speaks about legal technology and transformation. He is the founder Sideline and previously worked worked at Gilbert + Tobin and Axiom.
Also read: Cloud Practice Management Systems Review | John Duckett